The ethics of using AI in healthcare
—
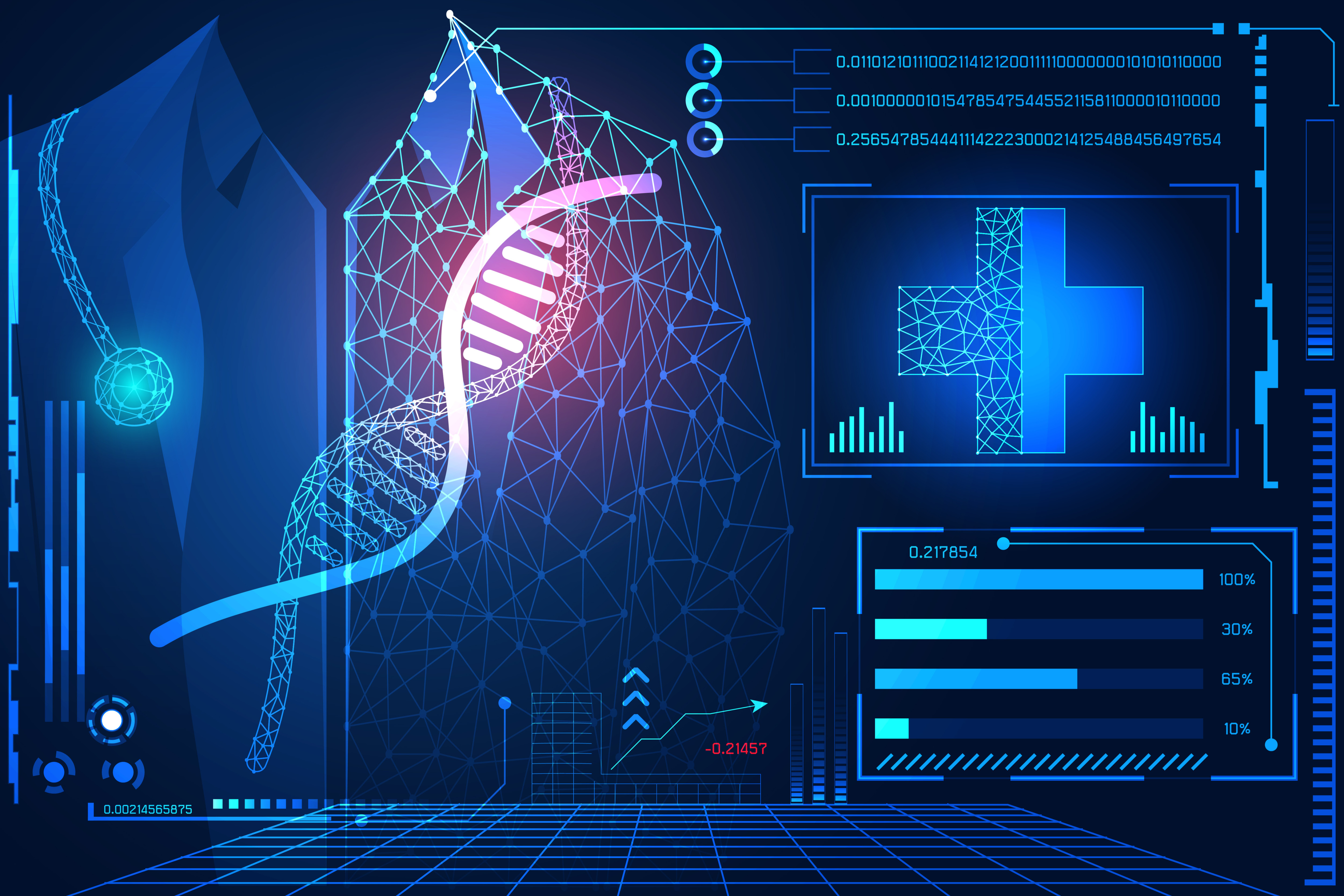
Introduction
The advent of Artificial Intelligence technology in healthcare has certainly created excitement, as it bears the possibility of providing better and more efficient care to billions of patients globally and improving clinical pathways through an interoperable healthcare ecosystem. The NHS in the UK, in particular, is still heavily reliant on outdated paper files and the majority of its IT systems are not based on open-standards, so the need for AI, innovation and disruptive technology in healthcare is urgent. With AI technologies being poised to transform and revolutionise the healthcare sector, we take a look at some of the ethical challenges that come with rolling it out.
Informed consent to use
Informed consent has become the primary standard for protecting patients’ rights and guiding the ethical practice of medicine and is based on the moral and legal premise of patient autonomy. In recent times, more people have been able to access and manage their own health records and control how their data is used. The NHS also has a national opt-out policy, whereby patients can opt out of having their health data used for research or planning purposes. A deadline of 30 September 2021 has been put in place to ensure that all health and care organisations comply with this policy1.
One of the most immediate challenges is integrating AI machine learning into clinical practice with informed consent, and how patient privacy is balanced with the safety and efficacy of AI learning. It also brings into question: under what circumstances must a clinician notify the patient that AI is being used? There is a lack of public understanding as to how patient data is used, and there is a desire from both patients and healthcare practitioners to be educated about this2.
This also raises questions about trust: can we rely on an app to diagnose us better than, or equally to, a doctor? To build trust, we must first understand why people are afraid of AI. Instead of dismissing people as Luddites, we need to take on board their concerns and ensure that they become part of the way forward. Values differ from country to country, and company to company. Whilst in China the use of face recognition technology is commonplace, people in the West are warier of this surveillance3. Public safety and ethical concerns relating to the use of AI is a central matter of interest for healthcare regulators and is something which is a top priority for rolling it out securely and beneficially.
Safety and transparency
A key challenge will be guaranteeing that AI and machine learning projects are developed and used in a way that is transparent and compatible with public interest, whilst stimulating and driving innovation in the sector4.The increased use of medical AI will endeavour to mitigate the levels of human error and misdiagnosis currently prevalent in routine healthcare. However, what if smart machines lead to new types of medical errors? And who would be held accountable for these errors if they arise? This is where Deep Learning – a branch of AI which is striving to replicate neural networks in the brain, and is seen as the future of AI – comes in to play. It is already being researched by various AI companies to determine whether or not machines are able to support decision making in a hospital by predicting what will likely happen to a patient.
People want transparency about the type of data shared, who it is used by and for what purpose, in addition to data security. It is the responsibility of AI developers and stakeholders to make sure this is always provided, as well as any shortcomings of the software being used (e.g. data bias)5.Technology and AI companies operating AI algorithms should be held accountable for system failures in the same way that other medical device or drug companies are held accountable6.
This can be achieved by ensuring that the used datasets and AI projects are reliable and valid; the better the training data, the better the AI will perform. Transparency creates trust amongst stakeholders, particularly patients and clinicians, which is vital to the successful implementation of AI in clinical practice.
Explainable AI is a set of tools and frameworks which help understand and interpret predictions made by machine learning models. With it, model performance can be improved and debugged, in addition to helping others understand the models’ behaviour7. XAI (explainable AI) is where machines understand the context and environment in which they operate, allowing them to create and build underlying explanatory models which then characterise real world situations.
Under GDPR, the regulation of algorithms, there exists a right to be given an explanation for an output of the algorithm. This is known as the explainability requirement and it is crucial for applications of AI and ML.
Bias
Biases can occur regarding ethnic origin, age, gender, and disabilities. The explanations for these biases can differ and are multi-layered. They can arise from the datasets themselves; fundamentally most bias comes down to the data that is used and the fact that it is predominantly collected within a context that is not representative i.e. most facial recognition datasets cover Caucasians, thus failing when dealing with BAME people. Biased AI could lead to false diagnoses and render treatments ineffective for certain subpopulations or groups of individuals. Some of these biases may, however, be resolved through an increasing availability and a wider variety of data.
Data privacy
Getting data right is key to truly harnessing the potential of AI in healthcare, especially for those seeking to use patient data; they must be able to demonstrate that they are adding value to the health of the patients whose data is being used. The power is also shifting from healthcare professionals to patients, whereby patients will be able to monitor their conditions, such as through remote monitoring capabilities, and medical histories. They will no longer be so in the dark, and this new era of transparency promises to make patient power the new norm.
The ‘Privacy by Design’ principle also comes in to play here, which constitutes protecting data through technology design and only using the absolute minimum set of data that is required for any given purpose. Behind this is the idea that data protection in data processing procedures is best adhered to when it is already integrated into the technology when it is being created8. Anonymisation and pseudonymisation are also crucial to data privacy. For anonymisation, any information which would make someone individually recognisable is removed thus making it impossible for a patient to be identified. Pseudonymisation is different to anonymisation, and is described under GDPR as “the processing of personal data in such a way that the data cannot be attributed to a specific data subject without the use of additional information, as long as additional information is kept separately and subject to technical and organisational measures to ensure non-attribution to an identified or identifiable individual”.
You can read more about securing data in a healthcare environment on our blog here.
Conclusion
It is essential that research focusing on these ethical challenges continues, drawing on the expertise of those who create and develop AI tools, those who will use and be impacted, and those who have knowledge and experience of addressing other major ethical challenges in healthcare9. It is of paramount importance that the voices of patients and their relatives are heard, and that their needs are kept in mind. It is only by developing tools that address real-world patient and clinician needs and tackle patient and clinician challenges that the opportunities of AI and related technologies can be maximised, whilst minimising the risks.
Ease of use and adoption of technology innovation is crucial to eliciting behaviour change, as humans are creatures of habit and we are often resistant to change – even if it’s for our benefit. AI must work in tandem with patients and healthcare professionals – not replace doctors as some people fear – to improve clinical pathways and the accuracy of diagnostics and treatment plans.
References
1. NHS – Compliance with the national data opt-out
3. Sanofi
4. Nuffield Council on Bioethics
5. NCBI
6. Reform
7. Google Cloud – Explainable AI
8. GDPR Privacy by Design – Intersoft Consulting