5 uses of machine learning in healthcare
—
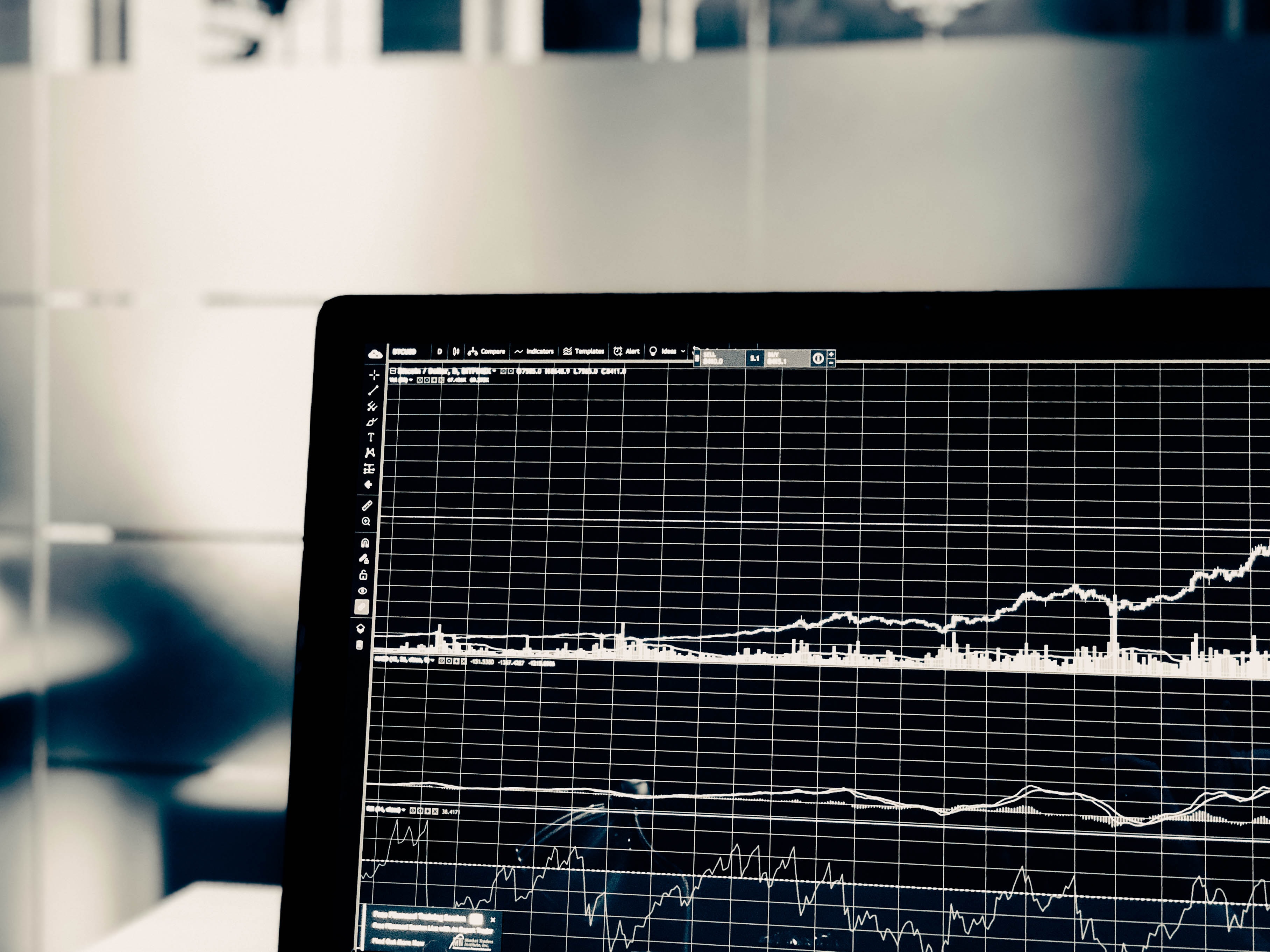
Machine learning, a subset of Artificial Intelligence, has seemingly endless possibilities and applications within the healthcare industry. At present, machine learning projects are helping establish and facilitate processes in hospitals, map and treat diseases, and personalise medical procedures.
The top five uses of machine-learning in healthcare:
1. Diagnostics
Diseases and ailments, particularly those that are hard to diagnose, are one of the key areas where machine learning comes into play, from cancers which are onerous to detect in the early stages to deciphering brain scans and genetic disease detection.
Algorithms provide instantaneous benefit to disciplines that have processes which can be standardised and repeated, for example those with large image data sets including cardiology, pathology and radiology. Machine learning can be taught to view images, pinpoint abnormalities, and direct attention to potential problem areas, therefore vastly improving the precision and efficiency of these processes through streamlining and overcoming human error, all whilst supplying a reliable objective opinion without any bias. As machine learning projects become more available and as they increase their explanatory capacity, AI-driven diagnostic processes will become the norm, aiding clinicians to provide quicker and more accurate disease diagnoses.
Machine learning occurs behind the scenes whenever a physician enters patient data (such as test results or symptoms) into their Electronic Health Record (EHR), providing the doctor with relevant information for making a diagnosis. The more data is collected, the more accurate and usable it will become through predictive analytics.
2. Therapeutics discovery and development
Research and development technologies, such as early-stage drug discovery and vaccine development, are a key area for machine learning clinical applications through learning and identifying patterns in data. Clinical trials also benefit from the use of machine learning, as the progression through the different phases can be accelerated, reducing both costs and time. Computational methods can take a mere few hours compared to months of real-life laboratory experiments, shaving time off outdated and slow procedures which can be allocated to more time efficient processes and further research, leading to a reduction in human workload. Real-time patient monitoring and reducing the amount of data-based errors in EHRs is also possible through machine learning technologies. AI is a powerful catalyst, and with the emergence of the Pfizer-BioNTech coronavirus vaccine, it has become evident that machine learning could become the new standard blueprint for forthcoming health solutions.
3. Patient data and record handling
Managing health records and ensuring they are up-to-date is an extensive process with technology only playing a role in allowing easier data entry up until recently. Generating smart health records which can offer clinical treatment suggestions and aid in diagnosis are now of paramount importance, with the crowdsourcing of extensive amounts of information, given consensually by patients, aiding researchers and practitioners on this journey. The value of machine learning in healthcare is its ability to digest vast sets of data beyond the level of human capabilities, analyse the evidence and provide accurate clinical insights, working in tandem with clinicians to enable them to make the best choices with regard to patient diagnoses and treatment plans.
4. Treatment and personalised medicine
Through combining predictive analytics with individual health, personalised treatments can be disseminated. This ultimately improves patient outcomes and satisfaction in addition to being useful for further research and enhanced disease assessment. At present, clinicians are limited to specific sets of diagnoses or make assumptions based on a patient’s personal history and available information, without inferring from further afield. The field of medicine is able to take great strides forward through the use of machine learning to present multiple treatment options and tailor them to the individual needs of each patient. In this vein, cutting-edge technologies including biosensors and other health measurement capabilities (such as HexOrthopaed and EWWD) will allow more data to become readily available.
5. Outbreak prediction
Monitoring and predicting epidemics around the globe, as we have seen through the current Covid-19 pandemic, can be done through machine learning and AI-based technologies and projects, forecasting outbreaks through artificial neural networks. This is of particular importance in developing countries where education systems and medical infrastructure are often less interconnected. Emerging and evolving diseases, for example, can be monitored, and real-time information provided on their status.
Benefits
Machine learning capabilities are central to the delivery of cost-effective, value-based, connected healthcare. They also allow for a more personalised and accurate approach to diagnosis and treatment by empowering patients to have more autonomy over their data and clinicians to have assistance that is of real benefit to them.
Helpfully, machine learning can be employed for predicting appointment no-shows, such as outpatient MRI scan appointments, which are a major problem and burden for healthcare centres. The prevention of this disruptive operational inefficiency, through the use of automatic prompts to attend appointments, is hugely beneficial in saving clinicians’ time and healthcare systems’ costs being wasted, allowing resources to be allocated and used more efficiently. The number of readmissions, rate of hospital acquired infections and length of stay in hospital can all be decreased through the use of machine learning, as clinicians will be given guidance as to how to lower these risks for their patients.
Chronic disease prediction and one-year mortality reduction can also be achieved through algorithmic predictions and insight by matching patients with the right methods for treatment, support and care.
Conclusion
Looking to the future, if used in ways that assist clinicians and enable ordinary citizens to take better care of their health by giving them the tools to do so, AI and machine learning have the capabilities to be transformational in directing efforts to address the health challenges we face today, such as an overall ageing population, unhealthy self-inflicted lifestyles and the rising occurrence of chronic diseases. A more connected healthcare system is currently being built, with the rate of progress continually increasing. The future of healthcare lies in machine learning and AI.